Inventory forecasting
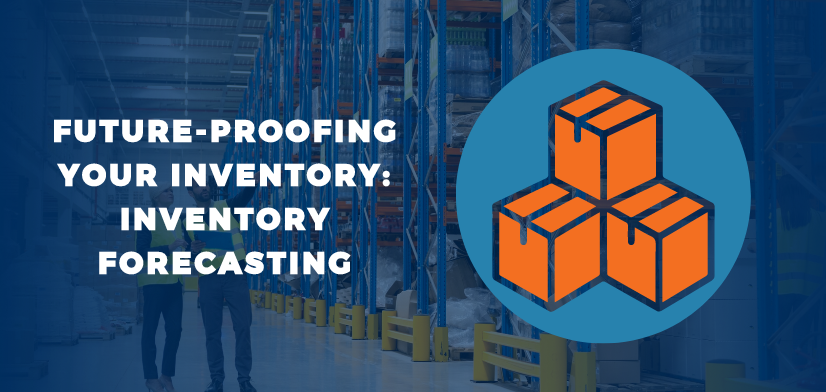
Inventory forecasting, also referred to as demand forecasting, is essential for retailers to predict future demand accurately.
Inventory forecasting, also known as demand forecasting, is the process of estimating future demand for products or services to determine the appropriate inventory levels to meet customer demand. It involves analyzing historical sales data, market trends, customer behavior, and other relevant factors to make informed predictions about future demand patterns.
Inventory forecasting is crucial for effective inventory management. By accurately predicting demand, retailers can ensure they have the right quantity of products on hand to meet customer needs. This prevents situations where customers are unable to find the products they want. Ultimately, forecasting helps retailers strike a balance between meeting customer demand and managing inventory costs. There are multiple ways you can approach inventory forecasting.
Quantitative Forecasting:
Quantitative forecasting relies on historical numeric data to predict future demand patterns. The accuracy of the forecast improves with a larger set of data. There are two commonly used methods for quantitative inventory forecasting:
Time-series forecasting: This method categorizes data points according to specific time periods, enabling the prediction of future seasonal patterns. Time-series forecasting involves employing mathematical models such as moving averages and exponential smoothing to identify patterns and make accurate predictions.
This approach utilizes real-time demand data obtained through point-of-sale (POS) systems or similar technologies to generate precise forecasts. By capturing up-to-date demand information, this method can provide accurate predictions.
Qualitative Forecasting:
Qualitative forecasting offers valuable insights when businesses have limited historical data for trend forecasting. Instead of relying on past sales data, this approach involves leveraging focus groups, surveys, and market research to develop forecasting models that predict future demand.
This method is very useful for businesses in their early stages or those that have changed their business model or products. Utilizing qualitative forecasting can also complement graphical and trend forecasting techniques, further enhancing the accuracy and reliability of predictions.
Trend Forecasting:
Trend forecasting utilizes sales data from the past and conducts market analysis to estimate future customer demand. It’s important to have enough sales data though, at least two years’ worth or more, to ensure a comprehensive analysis and accurate predictions. There are two main types of trend forecasting:
◆ Short-term forecasting, also known as seasonal forecasting, examines specific periods, typically within the next six months, to predict demand. This method is useful when the demand for products fluctuates due to seasonal factors, such as the increased demand for pool floats during the summer.
◆ Macro trends, also known as long-term forecasting, focus on analyzing broad indicators such as cultural or societal shifts that influence customer buying habits. This approach excludes seasonal impacts and irregularities that cannot be attributed to specific causes.
Graphical Forecasting:
Graphical forecasting involves analyzing historical data by graphing it to identify market trends and sales patterns. By visually representing the data, you can gain valuable insights and avoid overlooking important details hidden within the numbers.
The process is like trend forecasting, as it utilizes the same data. The key distinction is that graphical forecasting focuses on visually identifying patterns instead of reviewing numerical data as individual line items. If you prefer a visual approach to discern patterns, this method is ideal for you.
Incorporating one, or more, of these forecasting methods can enhance the reliability of your predictions, especially when dealing with seasonal fluctuations or shifts influencing customer buying habits. Ultimately, a well-executed forecasting process allows retailers to find the right balance between meeting customer demand and maintaining optimal inventory levels.